What are Predictions of Artificial Intelligence (AI) in Cybersecurity?
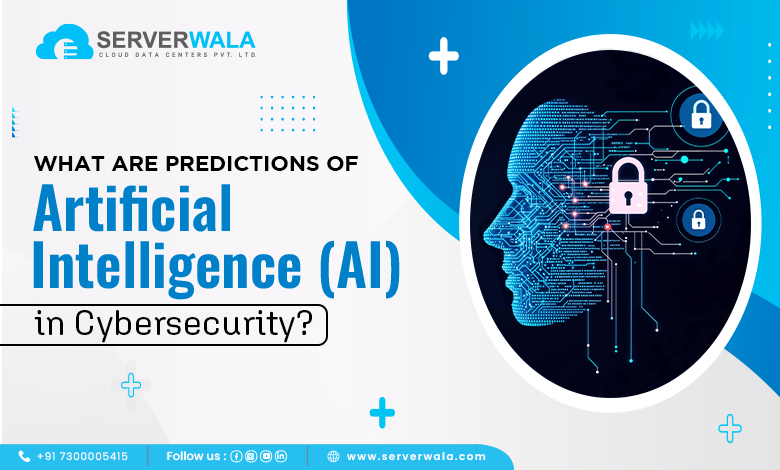
Introduction
Artificial Intelligence (AI) is revolutionizing how cybersecurity challenges are addressed. The rise of sophisticated cyber threats, incorporating ransomware, phishing, and advanced persistent threats (APTs), has exposed the limitations of traditional security measures. Static defenses and manual monitoring struggle to keep up with the speed as well as complications of contemporary cyberattacks.
AI steps in as a game-changer. It uses machine learning and data analytics to recognize & respond to threats quicker than human beings can. AI-driven systems examine huge volumes of data in real-time, recognizing anomalies and potential risks before they escalate. These capabilities enable organizations to shift from reactive to proactive cybersecurity approaches.
As cybercriminals advance their tactics, AI comes up alongside them, making defenses adaptive and intelligent. This article examines how AI is reshaping cybersecurity practices and explores its future potential in securing digital environments.
Purpose of the article: exploring future predictions of AI in cybersecurity
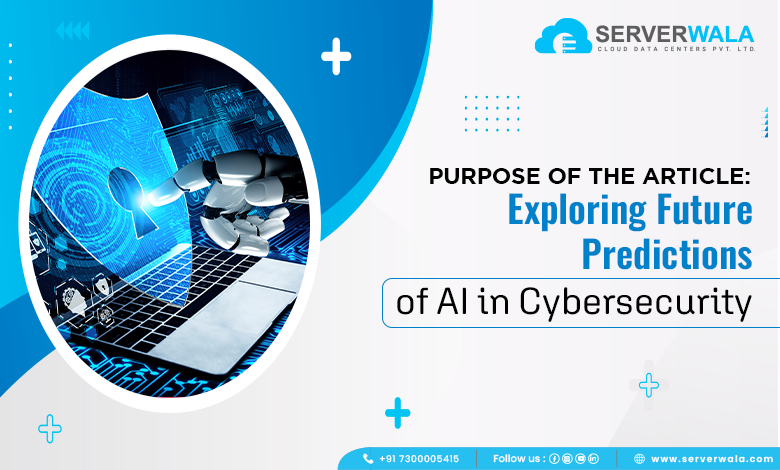
This article’s goal is to shed light on how AI is poised to transform cybersecurity in the coming years. Cyber threats are becoming increasingly complex and difficult to predict. To counter these, AI brings powerful tools that can adapt and respond in ways traditional systems cannot.
The discussion explores AI’s potential to replace outdated, manual threat recognition approaches. These methods often rely on static rules and human intervention, making them slow and limited in scope. AI, in opposite to this, uses machine learning to continuously evolve, identifying and mitigating risks more efficiently.
Another focus is on AI’s ability to learn from past breaches. By examining historical data, AI can uncover patterns and foretell future attack vectors. This predictive power helps organizations envision and prevent threats instead of just reacting to them.
AI’s role in securing IoT networks is another critical aspect. With billions of interconnected devices, IoT networks present a vast attack surface. AI-driven security systems monitor these networks, detect vulnerabilities, and respond to variances in real-time.
Finally, the article examines how AI will evolve alongside quantum computing. Quantum technology, while promising, introduces new cybersecurity challenges. AI will perform a significant role in devising quantum-resistant encryption and managing the risks held by quantum-based threats.
Understanding these trends allows businesses to prepare for both the prospects as well as challenges that will be in the forefront. By leveraging AI, entities can construct heavy defenses, guaranteeing a more safe digital future.
How AI will replace traditional, manual threat detection methods?
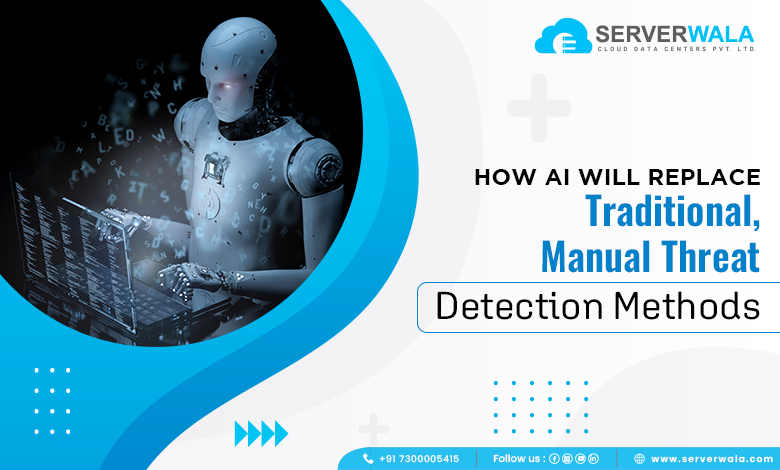
Traditional threat detection methods depend majorly on manual operations and predefined security rules. These methods are designed based on known threats, using signatures or pattern recognition to identify and block malicious activity. However, these approaches have significant limitations. They struggle against fast-evolving, sophisticated threats like zero-day vulnerabilities and advanced persistent threats (APTs). As cybercriminals continuously innovate, traditional systems fall short in detecting new or unknown threats.
AI is transitioning this arena by automating and enhancing threat detection. Using progressive machine learning algorithms, AI systems can continuously examine huge volumes of data in real-time. They process network traffic, user behavior, and even endpoint interactions to spot anomalies. Here’s how AI is reshaping threat detection:
- Real-Time Data Analysis: Traditional systems are limited by their reliance on static databases of known threats. In contrast, AI can analyze massive datasets in real-time. This allows it to detect subtle anomalies that might go unnoticed by humans or signature-based systems. For example, AI can identify unusual traffic patterns or abnormal login behaviors in a network, indicating a potential breach.
- Pattern Recognition and Adaptation: AI uses machine learning to recognize patterns and learn from new data. Unlike traditional approaches, which depend on human-defined rules, AI can take up evolving attack vectors. It can automatically update its algorithms to identify new types of attacks without needing human intervention. For instance, AI-driven systems in banking are now capable of detecting fraud by recognizing irregular spending behaviors, even if the fraud is not similar to previous patterns.
- Predictive Capabilities: The most resilient benefit of AI in threat detection is its foreseen ability. By examining old incidents, AI can forecast possible susceptibilities and attack strategies. For instance, ML models can be trained on previous cyberattack data to predict which systems might be targeted next. This enables entities to execute preventive strategies before an attack happens, unlike traditional methods that typically respond after an attack occurs.
- Reducing Response Times: Traditional threat detection often involves alerts that require human intervention for analysis, slowing down response times. AI systems can instantly detect and respond to threats by isolating compromised systems, blocking malicious IP addresses, or applying patches. This swift response is crucial in preventing extensive destruction. For example, AI-powered firewalls can immediately block an IP address that is associated with a DDoS attack, significantly reducing the time to mitigate the threat.
- Handling Unknown and Sophisticated Threats: AI excels at detecting unknown threats, such as zero-day attacks, that traditional methods may miss. Through techniques like anomaly detection, AI can flag suspicious behavior even if no known signature exists. This makes it invaluable for protecting against sophisticated attacks like fileless malware or polymorphic threats, which evolve rapidly to bypass conventional defenses.
- Continuous Improvement: Unlike traditional systems, AI-driven security solutions improve over time. As more data is operated, the system redefines its algorithms and becomes more accurate in threat detection. For example, AI models used in cybersecurity tools like CrowdStrike and Darktrace become more efficient at identifying new types of malware as they encounter more varied attack scenarios.
Enhancing security by enabling AI to learn from past breaches
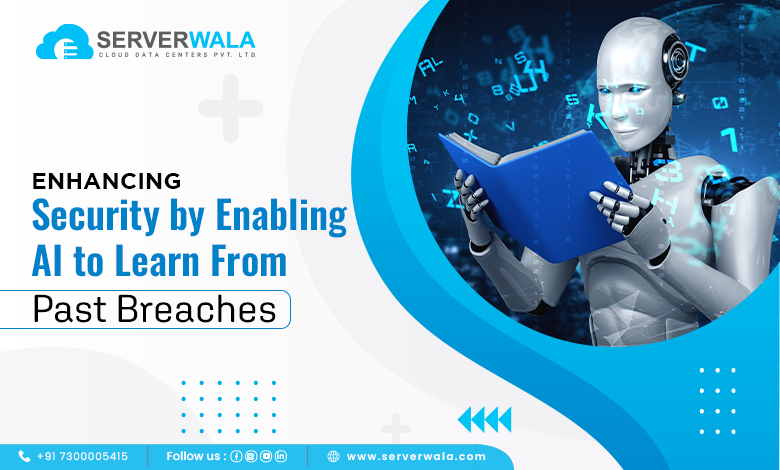
AI systems are highly effective at learning from historical data, particularly past security breaches. By apprehending data from old incidents, AI can recognize common vulnerabilities and attack tactics that were exploited. This consistent learning approach permits AI to refine its detection capabilities and improve over time.
Here’s how AI enhances security by learning from past breaches:
- Identifying Vulnerabilities: AI analyzes patterns from previous breaches to uncover common weaknesses in security systems. These could be software flaws, weak passwords, or inadequate network defenses. By recognizing these vulnerabilities, AI can help organizations patch them before attackers exploit them again. For instance, if a particular kind of ransomware has been thrived in the past, AI can prioritize defenses against that type of attack.
- Predicting Future Attacks: By studying past attack methods, AI can predict possible future threats. For example, if a breach occurred due to a specific kind of phishing email, AI can recognize similar email characteristics in real-time and flag them as suspicious. The AI system might even predict the likelihood of certain types of attacks, such as DDoS or social engineering attacks, based on historical data.
- Implementing Preventive Measures: AI-driven systems don’t just learn from past breaches; they also apply this knowledge to create proactive defense strategies. For example, if AI detects a pattern where certain vulnerable systems were targeted repeatedly, it can recommend strengthening these systems before any attack occurs. It can even automatically implement measures.
- Refining Detection Over Time: Machine learning models enhance with every new element of data they operate. This means that AI systems don’t just stop learning after a breach. They continuously adapt to new tactics, strategies, and procedures (TTPs) employed by attackers. Over time, this makes AI-driven security systems more precise and capable of recognizing contemporary kinds of threats.
- Adaptive Security Solutions: One of AI’s key advantages is its ability to provide adaptive security solutions. Unlike traditional static systems, which are often fixed in their approach, AI evolves as threats evolve. This guarantees that the system stays relevant as well as efficient in an ever-changing threat landscape.
For example, after a data breach where malware was introduced via a malicious attachment, AI can learn the tactics used and automatically start blocking similar attachments in future emails. This process allows businesses to stay ahead of attackers by making real-time adjustments to security protocols based on what has worked—and what hasn’t—in past attacks.
AI-Driven Security for the Internet of Things (IoT)
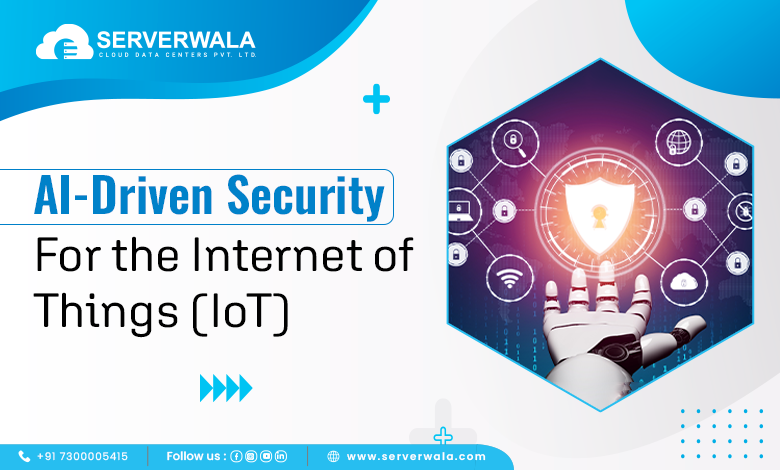
The rapid expansion of the Internet of Things (IoT) has constructed new challenges in cybersecurity. With zillions of devices linked to the internet, IoT networks present a vast attack surface. Traditional security measures often struggle to secure these networks due to their complexity and scale. AI renders a scalable & optimal solution to acknowledge these challenges.
Here’s how AI enhances IoT security:
- Monitoring for Anomalies: IoT devices, ranging from smart home gadgets to industrial sensors, generate a tremendous amount of data. AI can monitor these networks in real-time and detect any irregularities or unusual behavior. For instance, if a smart thermostat starts behaving erratically or if a sensor sends out a large number of requests unexpectedly, AI can detect these anomalies and flag them for further investigation. Early detection of abnormal behavior helps prevent potential cyberattacks before they spread.
- Detecting Unauthorized Access: IoT devices are frequently targeted by cybercriminals attempting to exploit weak security protocols. AI can help detect unauthorized access attempts or suspicious logins to IoT devices. By analyzing patterns of normal usage, AI can spot when a device is accessed from an unrecognized location or by an unfamiliar user. It can then initiate a response, such as isolating the device from the network or triggering an alert to system administrators.
- Isolating Compromised Devices: In the event of a breach, AI can automatically isolate compromised devices to prevent them from spreading malware or being used as entry points into other parts of the network. For example, if an AI system detects that a smart camera has been compromised, it can immediately disconnect it from the network, preventing the attacker from gaining access to other connected devices.
- Automating Software Updates and Patches: One of the key challenges in IoT security is keeping devices updated with the latest security patches. AI can automate the operation of updating software & applying patches to IoT devices. This helps ensure that devices are not left vulnerable to known exploits. For instance, if AI detects a newly discovered vulnerability in a connected device, it can push a software update or patch to prevent exploitation.
- Managing Device Vulnerabilities: Many IoT devices are manufactured with limited security features or have vulnerabilities that cybercriminals can exploit. AI can perform continuous vulnerability assessments across all connected devices. It can identify devices running outdated firmware, unsecured communications protocols, or weak passwords and alert administrators to take corrective actions.
- Creating a Seamless IoT Environment: AI’s ability to automate security tasks and provide real-time monitoring ensures that IoT networks remain secure while also functioning smoothly. As the number of connected devices grows, AI can scale its operations, providing security across thousands or even millions of devices without human intervention. This scalability makes AI an ideal solution for managing large, complex IoT ecosystems, especially in industries such as healthcare, manufacturing, and smart cities.
Example in Action
Consider a smart home system that administers everything from lights to safety cameras. AI can continuously monitor the entire network of connected devices for any abnormal behavior. If a camera starts sending unusual data or accessing the internet at unexpected times, the AI can immediately flag the camera, analyze its behavior, and isolate it from the network to prevent further damage. This automated approach to threat detection and response can prevent potential breaches from escalating into major incidents.
The Impact of Quantum Computing on AI in Cybersecurity
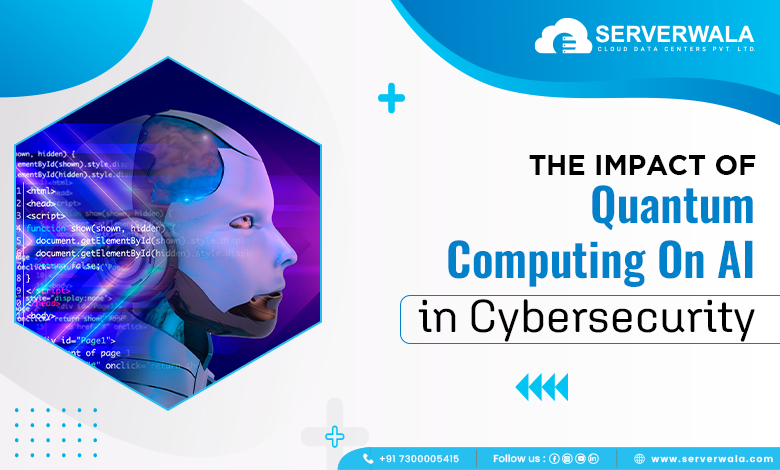
Quantum computing is revolutionizing the technological landscape by offering computational power far beyond what traditional computers can handle. However, this breakthrough also presents unique challenges, especially in the field of cybersecurity. Quantum computers have the potential to break existing encryption standards, which currently rely on mathematical algorithms that are difficult to solve with classical computing. For example, RSA encryption, widely used for securing communications, could be cracked by a enough resilient quantum system in a matter of seconds, while it would take classical systems several years to do the same.
Key Risks Posed by Quantum Computing:
- Breaking Encryption: The main threat quantum computing poses to cybersecurity is its ability to break existing cryptographic algorithms. Quantum algorithms, such as Shor’s Algorithm, could solve problems like factoring large numbers exponentially quicker than classical algorithms, rendering existing encryption approaches vulnerable. According to a study by the National Institute of Standards and Technology (NIST), it’s expected that quantum computers will be capable of breaking RSA and ECC (Elliptic Curve Cryptography) by the 2030s, with full-scale quantum computing breakthroughs possibly arriving as early as 2028.
- Weakening Digital Trust: Many of the trusted methods for secure online transactions—such as banking, e-commerce, and government communications—rely on these encryption techniques. If quantum computing can break these, it would undermine the foundation of secure digital communications. A 2023 report from McKinsey & Company highlights that quantum computing could render more than 90% of current public key cryptography techniques obsolete, prompting urgent needs for newer security protocols.
Despite these risks, AI is poised to play a crucial role in counteracting the potential threats posed by quantum computing.
How AI Acts as a Countermeasure: AI can develop and integrate quantum-resistant algorithms to create more secure encryption methods, anticipating quantum threats. Quantum-resistant algorithms use principles from quantum mechanics to create cryptographic protocols that are difficult for quantum computers to break. AI systems can design adaptive encryption strategies that evolve alongside advancements in quantum computing.
Here’s how AI can help:
- Quantum-Resistant Algorithms: AI can facilitate the development of encryption techniques that are resilient against quantum computing. By leveraging machine learning, AI can simulate quantum attacks on cryptographic systems, identifying weaknesses and proposing stronger encryption methods. For instance, lattice-based cryptography is one such quantum-resistant algorithm that AI could help optimize to protect against quantum attacks. Research from IBM and Google shows that AI can aid in identifying more efficient quantum-safe algorithms, which would be crucial in building secure systems in the quantum era.
- Faster Threat Detection and Response: AI-powered quantum computing could also enhance cybersecurity operations by enabling quicker threat detection and response. Quantum computing allows AI models to operation as well as examine large datasets much prompter than classical systems. This could significantly reduce the time it takes to identify and neutralize threats, such as cyberattacks on financial institutions or government systems. For example, a 2024 study from Stanford University demonstrated how quantum-enhanced machine learning algorithms can analyze patterns and anomalies in massive amounts of security data at a speed that classical systems cannot match, improving the accuracy and speed of threat identification.
- AI-Driven Quantum Security Systems: AI can work with quantum computing to build new, adaptive cybersecurity systems. These systems are able to learn from new data and constantly enhance their defense mechanisms. For example, AI can develop systems that predict where quantum computers might attack next, allowing organizations to proactively strengthen their defenses. As quantum computers become more capable, AI systems could use quantum machine learning techniques to analyze encrypted traffic patterns and detect vulnerabilities in real-time.
Quantum Computing and AI in Cybersecurity: Practical Applications
- Secure Communication Channels: AI can help build quantum-safe communication channels by applying quantum key distribution (QKD). QKD guarantees that any attempt to intercept the data will right away alter the encryption, making it detectable. This provides a secure way of transmitting sensitive data that even quantum computers cannot compromise.
- Enhanced Threat Modeling: AI, paired with quantum computing, can simulate potential cyberattack scenarios on a quantum scale. This could revolutionize threat modeling, where cybersecurity experts could visualize how different quantum algorithms might attack existing systems and develop countermeasures before such threats materialize.
- Automated System Security: AI can also be used to automatically update security protocols as quantum computing evolves. For example, AI could monitor ongoing developments in quantum research and quickly implement new encryption techniques as soon as they are proven secure. This would reduce the risk window for quantum-enabled attacks, ensuring systems remain ahead of the curve.
Looking to the Future
As quantum computing continues to evolve, AI will be essential in ensuring that cybersecurity systems remain resilient. The combination of quantum computing and AI has the potential to create a new era of cybersecurity—one where systems not only react to threats but anticipate and adapt to them in real time. However, businesses and governments will need to prepare for this new age by investing in both AI and quantum-safe technologies to safeguard their systems from emerging threats. According to Gartner, by 2028, over 50% of global enterprises will adopt quantum-safe encryption methods, with AI-driven solutions being at the forefront of these efforts.
Conclusion
AI is transitioning cybersecurity by rendering smarter, faster, and more adaptive solutions. Its ability to analyze large datasets, detect anomalies, and learn from past breaches ensures it stays ahead of evolving threats. Unlike static security systems, AI continuously improves its detection and prevention capabilities through machine learning.
Its impact extends across various domains. AI automates threat detection, enhances IoT security, and prepares systems for quantum-era challenges. It addresses gaps in traditional methods while offering scalability and precision. Moreover, its role in creating quantum-resistant encryption highlights its importance in future-proofing cybersecurity.
Businesses must recognize AI’s potential and integrate it into their security frameworks. By adopting AI-driven tools, entities can intensify their resilience against cyberattacks. The fusion of AI and cybersecurity is not just a trend but a necessity for protecting data, networks, and user trust in an increasingly digital world.